NWO LIFT
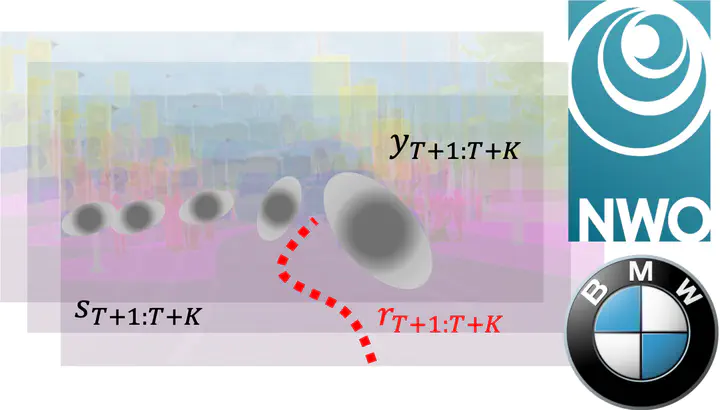
In today’s deep learning era in computer vision and machine learning, computers rival humans in what were once considered to be human-only tasks, with impressive results in object scene recognition, semantic segmentation and action classification. In this setting automotive companies, including BMW, Toyota and Tesla to name just three examples, have taken up the challenge to use deep learning technologies to design the next generation of vehicles, namely fully autonomous, self-driving cars. Current vehicles already enjoy conditional automation (level 3 out of 5), where the vehicle can drive autonomously for as long as no unexpected situations happen. However, the ultimate goal is to arrive at vehicles that drive fully autonomously (level 5 out of 5), where cars navigate without human intervention, avoiding collisions while driving comfortably and being socially aware of their surroundings. Going from conditional to full automation, however, is disproportionally hard, as the recent fatal accidents by the experimental Tesla and Uber autonomous vehicles have shown. Significant academic and industrial research efforts are necessary to reach autonomous vehicles with full automation levels that can predict and avoid collisions, while driving comfortably.
For research in collision avoidance, predicting future trajectories of traffic participants is of the utmost important. Unfortunately, current collision checkers operate under the assumption of a static world where objects do not move. Especially important are the most vulnerable traffic participants, like pedestrians, bicyclists and motorcyclists, who unlike cars, are highly manoeuvrable and cannot be modelled by standard techniques. Predicting the future trajectories of vulnerable traffic participants is crucial, as they run the highest risk of getting injured in accidents. In Germany alone 2.5 million traffic accidents occurred in 2016, including 65,000 heavy injuries and 3,000 fatalities, while in the Netherlands there were 613 fatalities in traffic accidents in 2017, 407 involving cyclists and motorcyclists. This proposal places special importance in predicting future trajectories of vulnerable traffic participants.