ERC Starting Grant
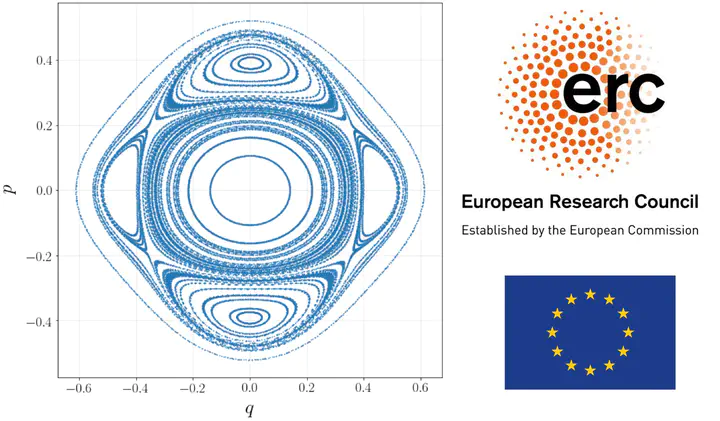
Visual artificial intelligence automatically interprets what happens in visual data like videos. Today’s research strives with queries like: “Is this person playing basketball?"; “Find the location of the brain stroke”; or “Track the glacier fractures in satellite footage”. All these queries are about visual observations already taken place. Today’s algorithms focus on explaining past visual observations. Naturally, not all queries are about the past: “Will this person draw something in or out of their pocket?"; “Where will the tumour be in 5 seconds given breathing patterns and moving organs?"; or, “How will the glacier fracture given the current motion and melting patterns?". For these queries and all others, the next generation of visual algorithms must expect what happens next given past visual observations. Visual artificial intelligence must also be able to prevent before the fact, rather than explain only after it. I propose an ambitious 5-year project to design algorithms that learn to expect the possible futures from visual sequences.
The main challenge for expecting possible futures is having visual algorithms that learn temporality in visual sequences. Today’s algorithms cannot do this convincingly. First, they are time-deterministic and ignore uncertainty, part of any expected future. I propose time-stochastic visual algorithms. Second, today’s algorithms are time-extrinsic and treat time as an external input or output variable. I propose time-intrinsic visual algorithms that integrate time within their latent representations. Third, visual algorithms must account for all innumerable spatiotemporal dynamics, despite their finite nature. I propose time-geometric visual algorithms that constrain temporal latent spaces to known geometries.
EVA addresses fundamental research issues in the automatic interpretation of future visual sequences. Its results will serve as a basis for ground-breaking technological advances in practical vision applications.